Facts, Attributes, and Metrics
Facts, Attributes and Metrics
What are they? How do they work? – https://help.tableau.com/current/pro/desktop/en-us/datafields_typesandroles.htm
What are Facts and Attributes?
Facts and Attributes are two different types (classes) of data columns. They refer to what they represent or model in real-life. are just the roles that Inzata assigns to your data automatically.
Every column in your data is either a Fact or an Attribute.
By default, Inzata automatically assigns a Fact or Attribute role to every column based on that column’s properties and context when the data is loaded, but you can switch this role for any column manually, if you wish.
Why assign roles to my data?
Your ultimate goal is to analyze that data to answer questions. Your data are going to have different roles to play in your Inzata Data Warehouse! Remember, your data is merely there to tell a story about something that happened in real life. And in real life, there are people, places and things (Sometimes called ‘entities’) that interact and relate to one another (‘relationship’) and sometimes do things (‘activities’).
Attributes contain qualitative values (such as names, dates, or geographical data). You can use attributes to categorize, segment, filter and reveal the details in your data. Attributes affect the level of detail in the view. Attributes can be either alpha, or numeric, or a combination of both. Attributes can also change over time. An example of a numeric Attribute would be something like Zip Code or Phone Number.
Facts on the other hand contain quantitative (or numeric) values. Facts are a record of something that happened in the real world. They never change. They’re a standing record of something that happened in the past. Examples of Facts would include the details about a product sale such as “Transaction Total” or “Purchase Quantity”. Other examples would include a column called “Temperature” containing temperature readings taken every hour.
Questions for review – Attributes and Facts
- Why do we not want facts to change over time?
- Why would we want to change an attribute? Give an example of an attribute and why you might change it.
- Classify the following Columns into Facts or Attributes (Best guess is fine!)
- Zip Code 6. College Major
- Time 7. Engine Type
- Temperature 8. Purchase Amount
- Eye Color 9. Sales Tax Collected
- Test Score 10. City Sales Tax Rate
What are Metrics?
- Metrics are a way to make Facts more useful! Metrics are formulas that aggregate your facts, turning them into new numbers, like Sum, Average or Top-100. If you’ve ever used the =SUM formula in Excel, you’ve created a Metric!
- Facts, all by themselves, just aren’t very useful in Business Intelligence. Having the purchase amount of a single transaction doesn’t tell you much about the health of your business.
- That’s where Analytics platforms like Inzata come in. Inzata lets you take huge quantities of Facts, and through something called aggregation, turn them into insights that provides a lot more value.
- By aggregating the facts into a Sum total or an Average, or a Count, we can learn a lot about our how our business is doing.
- To take it a step further, when we combine Metrics with Attributes, we can get something like “Total Sales broken down by Region”, we’re taking a fact (“Sales”), then the “Total Sales” metric aggregates it, and finally the “by Region” attribute gives us a nice table showing sales broken down by region. Quite useful!
- Metrics and Attributes are the primary building blocks of reports and dashboards in Inzata! Every dashboard you create will be made up of Metrics and Attributes. How you mix these elements together will determine what business questions your reports and dashboards can answer.
Questions for review – Metrics: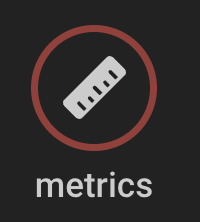
- What is the process that allow Metrics to make Facts more valuable?
- Why are facts by themselves not very useful?
- What is something that all Metrics need to have?
- Name 3 types of aggregation functions.
- Metrics + ________ are the primary building blocks of reports and Dashboard in Inzata.
What are some common Data Analysis mistakes?
- Not understanding what your data represents.
- Not having a clear business problem or question you’re trying to answer.
- Using the wrong type of visual for the story you’re trying to tell.
- Not addressing missing or null values or checking for bad data.
- Looking at the wrong time period or not aggregating consistently.
- Not doing any quality checks.
- Not communicating properly with the appropriate stakeholders.
- Reaching a conclusion before analyzing the data, then skewing analysis to support that conclusion.
- Skipping the step of exploring and understanding the data before diving into complex analyses (exploratory data analysis).